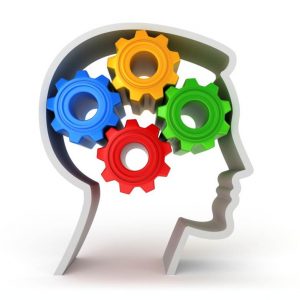
Automation and Jobs
A report by Oxford Martin School, University of Oxford (The Report), has examined the susceptibility of jobs to computerization. The impact of computerisation on jobs (labour market) is well-established. It is documented that there will be a decline in routine -intensive occupations, that is, occupations mainly consisting of task following well-defined procedures that can easily be performed by sophisticated algorithms.
At the same time, with falling prices of computers, problem-solving skills are becoming productive, which explains substantial employment growth in occupations involving cognitive tasks where skilled labour has a comparative advantage. According to Brynjolfsson and McAfee (2011), technological innovation is still increasing with more sophisticated technologies disrupting labour by making workers and employees redundant.
According to Autor, et al. (2003) workplace tasks can be categorized as follows:
- Routine versus non-routines tasks, and
- Manual versus cognitive tasks.
In short, routine tasks are defined as tasks that follow explicit rules that can be accomplished by machines while, while non-routine tasks are not sufficiently well understood in computer codes. Each of these task categories can, in turn, be of either manual or cognitive in nature, that is, they relate to physical labour or knowledge work.
Perception and Manipulation Tasks
Robots are still unable to match the depth and breadth of human perception. While basic geometric identification is reasonably mature, enabled by the rapid development of sophisticated sensors and lasers, significant challenges remain for more complex perception tasks, such as identifying objects and their properties in a cluttered field of view. As such, tasks that relate to an unstructured work environment can make jobs less susceptible to computerisation. The difficulty of perception has ramifications for manipulation tasks. This is, in particular, the handling of irregular objects, for which robots are yet to reach human level of aptitude.
A related challenge is failure recovery, that is, identifying and rectifying the mistakes of the robot when it has, for example, dropped an object. Manipulation is also limited by the difficulties of planning out the sequence of actions required to move an object form one place to another.
The main challenges to robotic computerization, perception and manipulation, thus largely remain and are unlikely to be fully resolved in the next decade or two.
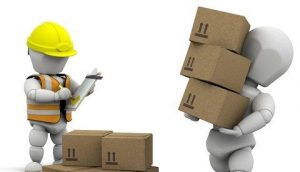
Creative and Intelligence Tasks
The psychological processes underlying human creativity are difficult to specify. According to Borden (2003), creativity is the ability to come up with ideas or artifacts that are novel and valuable. Ideas, in a broader sense, include concepts, poems, musical compositions, scientific theories, cooking recipes and jokes, whereas artifacts are objects such as paintings, sculptures, machinery and pottery. One process of creating ideas (and similarly artifacts) involves making unfamiliar combinations of familiar ideas, requiring a rich store of knowledge. The challenge here is to find some reliable means of arriving at combinations that “make sense.”
It seems unlikely that occupations requiring a high degree of creative intelligence will be automated in the next decades.
Social Intelligence Tasks
Human social intelligence is important in a wide range of work tasks, such as those involving negotiations, persuasion and care. While algorithms and robots can reproduce some aspects of human social interaction, the real-time recognition of natural human emotion remains a challenging problem, and the ability to respond intelligently to such inputs is even more difficult. Even simplified versions of typical social tasks prove difficult for computers, as is the case in which social interaction is reduced to pure text.
The authors of the Oxford Martin School’s report noted that while sophisticated algorithms and development in MR, building upon big data now allow many non-routine tasks to be automated, occupations that involve complex perception and manipulation tasks, creative intelligence tasks, and social intelligence tasks are unlikely to be substituted by computer capital over the next decades or two.
The probability of an occupation being automated can thus be described as a function of these task characteristics.
Measuring Impact of Computerisation
The Report, using 702 detailed occupation information of the US Labour Department’s Standard Occupation Classification (SOC), has developed a model to measure the impact of computerization of various types of occupations.
Table 1 shows the top 20 occupations that are least-computerisable , while Table 2 shows the top 20 occupations that are most-computerisable.
Table 1: Top 20 Least-Computerisable
Rank | Probability | SOC Code | Occupation |
1 | 0.0028 | 29-1125 | Recreational Therapists |
2 | 0.003 | 49-1011 | First-Line Supervisors of Mechanics, Installers and Repairers |
3 | 0.003 | 11-9161 | Emergency Management Directors |
4 | 0.0031 | 21-1023 | Mental Health and Substance Abuse Social Workers |
5 | 0.0033 | 29-1181 | Audiologists |
6 | 0.0035 | 29-1122 | Occupational Therapists |
7 | 0.0035 | 29-2091 | Orthotists and Prosthetists |
8 | 0.0035 | 21-1022 | Healthcare Social Workers |
9 | 0.0036 | 29-1022 | Oral and Maxillofacial Surgeons |
10 | 0.0036 | 33-1011 | First-Line Supervisors of Fire Fighting and Prevention Workers |
11 | 0.0039 | 29-2031 | Dietitians and Nutritionists |
12 | 0.0039 | 11-9081 | Lodging Managers |
13 | 0.004 | 27-2032 | Choreographers |
14 | 0.0041 | 41-9031 | Sales Engineers |
15 | 0.0042 | 29-1060 | Physicians and Surgeons |
16 | 0.0042 | 25-9031 | Instructional Coordinators |
17 | 0.0043 | 19-3039 | Psychologists and, All Others |
18 | 0.0044 | 33-1012 | First-Line Supervisors of Police and Detectives |
19 | 0.0044 | 29-1021 | Dentists, General |
20 | 0.0044 | 25-2021 | Elementary School Teachers |
Table 2: Top 20 Most-Computerisable
Rank | Probability | SOC Code | Occupation |
1 | 0.99 | 41-9041 | Telemarketers |
2 | 0.99 | 23-2093 | Title Examiners, Abstractors and Searchers |
3 | 0.99 | 51-6051 | Sewers Hand |
4 | 0.99 | 15-2091 | Mathematical Technicians |
5 | 0.99 | 13-2053 | Insurance Underwriters |
6 | 0.99 | 49-9064 | Watch Repairers |
7 | 0.99 | 43-5011 | Cargo and Freight Agents |
8 | 0.99 | 13-2082 | Tax Preparers |
9 | 0.99 | 51-9151 | Photographic Process Workers |
10 | 0.99 | 43-4141 | New Account Clerks |
11 | 0.99 | 25-4031 | Library Technicians |
12 | 0.99 | 43-9021 | Data Entry Keyers |
13 | 0.98 | 51-2093 | Timing Device Assemblers and Adjusters |
14 | 0.98 | 43-9041 | Insurance Claims and Policy Processing Clerks |
15 | 0.98 | 43-4011 | Brokerage Clerks |
16 | 0.98 | 43-4151 | Order Clerks |
17 | 0.98 | 13-2072 | Loan Officers |
18 | 0.98 | 27-2023 | Umpires, Referees and Other Sport Officials |
19 | 0.98 | 43-3071 | Tellers |
20 | 0.98 | 51-9194 | Etchers and Engravers |
Please see the whole list of 702 occupations in Appendix of Oxford Martin School’s Report.
Highlights
The Report’s main conclusions are as follows:
- It distinguishes high, medium and low risk occupations, depending on their probability of computerisation. It makes no attempt to estimate the number of jobs that will actually be automated, and focus on potential job automatability over some unspecified number of years.
- It predicts that most workers in transportation and logistics occupations, together with the bulk of office and administrative support workers, and labour in production occupations, are at risk.
- It provides evidence that wages and educational attainment exhibit a strong negative relationship with the probability of computerization.
- It implies that as technology races ahead, low-skill workers will reallocate to tasks that are non-susceptible to computerization, that is, tasks requiring creative and social intelligence. For workers to win the race, however, they will have to acquire creative and social skills.
Reference:
- Carl Benedict Frey, and Micheal A. Osborne (20130, The future employment: How susceptible are jobs to computerisation. Working Paper, Oxford Martin School, University of Oxford.
- Brynjolfsson and E. McAffe (2011). Race against the machine: How the digital revolution is accelerating innovation, driving productivity, and irreversibility transforming employment and economy. Digital Frontiers Press, Lexington, MA.
- A. Boden (2003). The creative mind: Myths and mechanisms. Routledge.
- Autor, F. Levy and R. J. Murnane (2003). The skill content of recent technological change: Am empirical exploration. The Quarterly Journal of Economics. Vol. 118, no.4, pp. 1279-1333.